Alignment Can Be The ‘clean Energy’ Of Ai
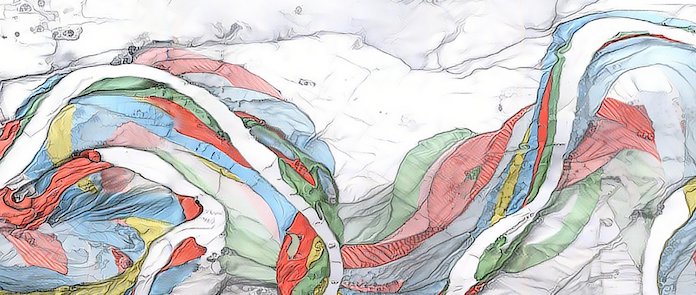
Not all that long ago, the idea of advanced AI in Washington, DC seemed like a nonstarter. Policymakers treated it as weird sci‐fi-esque overreach/just another Big Tech Thing. Yet, in our experience over the last month, recent high-profile developments—most notably, DeepSeek's release of R1 and the $500B Stargate announcement—have shifted the Overton window significantly.
For the first time, DC policy circles are genuinely grappling with advanced AI as a concrete reality rather than a distant possibility. However, this newfound attention has also brought uncertainty: policymakers are actively searching for politically viable approaches to AI governance, but many are increasingly wary of what they see as excessive focus on safety at the expense of innovation and competitiveness. Most notably at the recent Paris summit, JD Vance explicitly moved to pivot the narrative from "AI safety" to "AI opportunity"—a shift that the current administration’s AI czar David Sacks praised as a "bracing" break from previous safety-focused gatherings.
Sacks positions himself as a "techno-realist," gravitating away from both extremes of certain doom and unchecked optimism. We think this is an overall-sensible strategic perspective for now—and also recognize that halting or slowing AI development at this point would, as Sacks puts it, “[be] like ordering the tides to stop.”[1] The pragmatic question at this stage isn't whether to develop AI, but how to guide its development responsibly while maintaining competitiveness. Along these lines, we see a crucial parallel that's often overlooked in the current debate: alignment research, rather than being a drain on model competitiveness, is likely actually key to maintaining a competitive edge.
Some policymakers and investors hear "safety" and immediately imagine compliance overhead, slowdowns, regulatory capture, and ceded market share. The idea of an "alignment tax" is not new—many have long argued that prioritizing reliability and guardrails means losing out to the fastest (likely-safety-agnostic) mover. But key evidence continues to emerge that alignment techniques can enhance capabilities rather than hinder them (some strong recent examples are documented in the collapsible section below).[2]
This dynamic—where supposedly idealistic constraints reveal themselves as competitive advantages—would not be unique to AI. Consider the developmental trajectory of renewable energy. For decades, clean power was dismissed as an expensive luxury. Today, solar and wind in many regions are outright cheaper than fossil fuels—an advantage driven by deliberate R&D, policy support, and scaling effects—meaning that in many places, transitioning to the more ‘altruistic’ mode of development was successfully incentivized through market forces rather than appeals to long-term risk.[3]
Similarly, it is plausible that aligned AI, viewed today as a costly-constraint-by-default, becomes the competitive choice as soon as better performance and more reliable and trustworthy decisions translate into real commercial value. The core analogy here might be to RLHF: the major players racing to build AGI virtually all use RLHF/RLAIF (a [clearly imperfect] alignment technique) in their training pipelines not because they necessarily care deeply about alignment, but rather simply because doing so is (currently) competitively required. Moreover, even in cases where alignment initially imposes overhead, early investments will bring costs down—just as sustained R&D investment slashed the cost of solar from $100 per watt in the 1970s to less than $0.30 per watt today.[4]
(10 recent examples of alignment-as-competitive-advantage)
A growing body of research demonstrates how techniques often framed as “safety measures” can also globally improve model performance.
1. Aligner: Efficient Alignment by Learning to Correct (Ji et al., 2024)
- Core finding: Aligner, a small plug-in model trained to correct a base LLM’s mistakes by learning the residuals between preferred and dispreferred answers, dramatically improves the base model’s helpfulness, harmlessness, and honesty.
- Global benefit: demonstrates that a single well-trained critique model can upgrade many systems’ safety and quality simultaneously (even without modifying the original LLM), rendering this alignment technique an efficiency gain rather than a cost.
2. Shepherd: A Meta AI Critic Model (Wang et al., 2023)
- Core finding: introduces Shepherd, a 7B-parameter model finetuned to give feedback, identify errors, and suggest fixes in other models’ outputs so well that GPT-4 and human evaluations significantly prefer Shepherd’s critiques over those from much larger models.
- Global benefit: demonstrates that investing in alignment-focused tools (like a dedicated critic model) can elevate overall system performance: even a smaller aligned model can drive better results from a larger model by refining its answers, effectively amplifying quality without needing to scale up the main model.
3. Zero-Shot Verification-Guided Chain of Thought (Chowdhury & Caragea, 2025)
- Core finding: demonstrates that an LLM can use a zero-shot self-verification mechanism — breaking its reasoning into steps with a special COT STEP prompt and then using its own internal verifier prompts to check each step — to improve accuracy on math and commonsense questions without any fine-tuned verifier or handcrafted examples.
- Global benefit: suggests that alignment can be embedded into the reasoning process itself (via the model checking its own chain-of-thought), which enhances correctness and reliability at inference time without extra training, showing that alignment techniques can directly translate to better performance even in zero-shot settings.
4. Multi-Objective RLHF (Mukherjee et al., 2024)
- Core finding: uses a hypervolume maximization approach to obtain a diverse set of LLM policies that achieve Pareto-optimal alignment across conflicting objectives (helpfulness, harmlessness, humor, etc.), outperforming baseline methods on all these alignment measures.
- Global benefit: demonstrates that alignment can be handled for many criteria concurrently without sacrificing one for another, providing a way to make models simultaneously safer and more useful rather than trading off capability for alignment.
5. Mitigating the Alignment Tax of RLHF (Lin et al., 2023)
- Core finding: techniques that average the weights of a model before and after RLHF fine-tuning (model merging) yields the best balance between maintaining the model’s original capabilities and achieving alignment, outperforming more complex forgetting-mitigation techniques on the alignment-vs-performance Pareto curve.
- Global benefit: merging models can maximize alignment gains with minimal loss of pre-trained knowledge.
6. RAG-Reward: Optimizing RAG with Reward Modeling and RLHF (Zhang et al., 2025)
- Core finding: presents RAG-Reward, a large-scale preference dataset and benchmark for evaluating retrieval-augmented LLMs. The authors train a reward model on this dataset and use it in RLHF, significantly improving factual accuracy and reducing hallucinations in RAG-generated responses.
- Global benefit: By integrating reward feedback directly into the knowledge retrieval and generation process, the LLM becomes both more trustworthy and more effective at answering questions.
- Core finding: finetuning models to critique incorrect solutions (instead of imitating correct ones) yields superior mathematical reasoning performance compared to standard supervised fine-tuning and matching the results of models trained on orders-of-magnitude more data, with only ~50K training examples and about an hour of training.
- Global benefit: training models with an alignment-focused objective (critical feedback rather than pure imitation) can make them both smarter and more efficient to train, as the CFT models reached top-tier performance using just 50K examples and minimal compute (versus competitors needing 140× more).
8. Step-KTO: Optimizing Mathematical Reasoning through Stepwise Binary Feedback (Lin et al., 2025)
- Core finding: introduces training with binary correct/incorrect feedback at each reasoning step (and at the final answer), which leads LLMs to follow logical reasoning paths and significantly improves their accuracy on challenging math benchmarks.
- Global benefit: demonstrates that aligning the model’s intermediate reasoning with correctness checks not only makes its process more interpretable but also enhances end performance—i.e., the model doesn’t just behave better; it actually solves problems more successfully when guided by stepwise feedback.
9. Feature Guided Activation Additions (Soo et al., 2025)
- Core finding: introduces a new technique for steering LLM outputs by injecting carefully chosen activation vectors (derived from SAE features), which yields more precise and interpretable control over model behavior than prior activation-steering methods.
- Global benefit: using alignment-based interventions at the feature level, developers can guide models to desired outputs without retraining, improving reliability and safety in specific contexts as a gain in capability (the model can follow instructions more exactly) rather than as a restriction.
10. Evolving Deeper LLM Thinking (Lee et al., 2025)
- Core finding: introduces an approach where the LLM iteratively evolves its own answers (generating, recombining, and refining candidate solutions under a fixed compute budget), achieving far higher success rates on complex planning tasks than traditional one-shot or simple iterative methods at the same inference cost.
- Global benefit: illustrates that aligning the inference process itself—essentially encouraging the model to self-optimize and self-refine its solutions—can dramatically improve outcomes without any extra model training.
This research trend clearly indicates alignment-inspired techniques can translate directly into more competent models, which can in turn render short-term competitiveness gains—in addition to a long-term hedge against existential threats.[5]
Certainly, no one claims every alignment technique will yield a “negative tax.” But even so, there now seems to be enough empirical evidence to undermine the blanket assumption that safety is always a drain. And if we hope to see alignment become standard practice in model development—similar to how robust QA processes became standard in software—these examples can serve as proof points that alignment work is not purely altruistic overhead.
Scaling neglected alignment research
The business case for investing in alignment research has become increasingly compelling. As frontier AI labs race to maintain competitive advantages, strategic investment in alignment offers a path to both near-term performance gains and long-term sustainability. Moreover, there's a powerful network effect at play: as more organizations contribute to alignment research, the entire field benefits from accelerated progress and shared insights, much like how coordinated investment in renewable energy research helped drive down costs industry-wide.
And even with promising new funding opportunities, far too many projects remain starved for resources and attention. Historically, major breakthroughs—from jumping genes to continental drift to ANNs—often emerged from overlooked or “fringe” research. Alignment has its own share of unorthodox-yet-promising proposals, but they can easily languish if most funding keeps flowing to the same small cluster of relatively “safer” directions.
One path forward here is active government support for neglected alignment research. For instance, DARPA-style programs have historically funded big, high-risk bets that mainstream funders ignored, but we can imagine any robust federal or philanthropic effort—grants, labs, specialized R&D mandates—structured specifically to test promising alignment interventions at scale, iterate quickly, and share partial results openly.
This kind of parallelization is powerful and necessary in a world with shortened AGI timelines: even if, by default, the vast majority of outlier hunches do not pan out, the handful that show promise could radically reduce AI's capacity for deceptive or hazardous behaviors, and potentially improve base performance. At AE Studio, we've designed a systematic approach to scaling neglected alignment research, creating an ecosystem that rapidly tests and refines promising but underexplored ideas. While our early results have generated promising signals, scaling this research requires broader government and industry buy-in. The U.S. should treat this as a strategic advantage, similar to historical investments in critical defense and scientific initiatives. This means systematically identifying and supporting unconventional approaches, backing high-uncertainty but high-upside R&D efforts, and even using AI itself to accelerate alignment research. The key is ensuring that this research is systematically supported, rather than tacked on as a token afterthought—or ignored altogether.
Three concrete ways to begin implementing this vision now
As policymakers grapple with how to address advanced AI, some propose heavy-handed regulations or outright pauses, while others push for unbridled acceleration.
Both extremes risk missing the central point: the next wave of alignment breakthroughs could confer major market advantages that are completely orthogonal to caring deeply about existential risk. Here are three concrete approaches to seize this opportunity in the short-term:
- Incentivizing Early Adoption (Without Penalizing Nonadoption): Consider analogies like feed-in tariffs for solar or the R&D tax credits for emerging biotech. Government players could offer compute credits, direct grants, or preferential contracting to firms that integrate best-in-class alignment methods—or that provide open evidence of systematically testing new safety techniques.
- Scale Up “Fighting Fire with Fire” Automation: Instead of relying solely on human researchers to keep up with frontier models, specialized AI agents should be tasked with alignment R&D and rapidly scaled as soon as systems/pipelines are competent enough to do contribute real value here (frontier reasoning models with the right scaffolding probably clear this bar). Despite its potential, this approach remains surprisingly underleveraged both within major labs and across the broader research community. Compared to the costs of human research, running such systems with an expectation that even ~1% of their outputs are remotely useful seems like a clearly worthwhile short-term investment.
- Alignment requirements for HPC on federal lands: there are promising proposals to build ‘special compute zones’ to scale up AI R&D, including on federal lands. One sensible policy following up on this might be requiring HPC infrastructure on federal lands (or infra otherwise funded by the federal government) to allocate a percentage of compute resources to capability-friendly alignment R&D.
Such measures will likely yield a virtuous cycle: as alignment research continues to demonstrate near-term performance boosts, that “tax” narrative will fade, making alignment the competitively necessary choice rather than an altruistic add-on for developers.
A critical window of opportunity
In spite of some recent comments from the VP, the Overton window for advanced AI concerns in DC seems to have shifted significantly over the past month. Lawmakers and staff who used to be skeptical are actively seeking solutions that don’t just boil down to shutting down or hampering current work. The alignment community can meet that demand with a credible alternative vision:
- Yes, advanced AI poses real risks;
- No, on balance, alignment is not a cost;
- We should invest in neglected AI alignment research, which promises more capable and trustworthy systems in the near-term.
Our recent engagements with lawmakers in DC indicate that when we focus on substantive discussion of AI development and its challenges, right-leaning policymakers are fully capable of engaging with the core issues. The key is treating them as equal partners in addressing real technical and policy challenges, not talking down to them or otherwise avoiding hard truths.
If we miss this window—if we keep presenting alignment as a mandatory "tax" that labs must grudgingly pay rather than a savvy long-term investment in reliable frontier systems—then the public and policy appetite for supporting real and necessary alignment research may semi-permanently recede. The path forward requires showing what we've already begun to prove: that aligned approaches to AI development may well be the most performant ones.
- ^
Note that this may simply reflect the natural mainstreaming of AI policy: as billions in funding and serious government attention pour in, earlier safety-focused discussions inevitably give way to traditional power dynamics—and, given the dizzying pace of development and the high variance of the political climate, this de-emphasis of safety could prove short-lived and things like a global pause may eventually come to be entirely plausible.
- ^
At the most basic level, models that reliably do what developers and users want them to do are simply better products. More concretely—and in spite of its serious shortcomings as an alignment technique—RLHF still stands out as the most obvious example: originally developed as an alignment technique to make models less toxic and dangerous, it has been widely adopted by leading AI labs primarily because it dramatically improves task performance and conversational ability. As Anthropic noted in their 2022 paper, "our alignment interventions actually enhance the capabilities of large models"—suggesting that for sufficiently advanced AI, behaving in a reliably aligned way may be just another capability.
It is also worth acknowledging the converse case: while it is true that some capabilities research can also incidentally yield alignment progress, this path is unreliable and indirect. In our view, prioritizing alignment explicitly is the only consistent way to ensure long-term progress—and it’s significantly more likely to reap capabilities benefits along the way than the converse. - ^
Take the illustrative case of Georgetown, Texas: in 2015, the traditionally conservative city transitioned to 100% renewable energy—not out of environmental idealism, but because a straightforward cost–benefit analysis revealed that wind and solar offered significantly lower, more stable long-term costs than fossil fuels.
- ^
These kinds of trends also reflect a broader economic transition over the course of human history: namely, from zero-sum competition over finite resources to creating exponentially more value through innovation and cooperation.
- ^
Of course, methods like these are highly unlikely to be sufficient for aligning superintelligent systems. In fact, improving current capabilities can create new alignment challenges by giving models more tools to circumvent or exploit our oversight. So while these techniques deliver real near-term benefits, they do not eliminate the need for deeper solutions suited to stronger AI regimes.
Discuss