Computers, Vol. 14, Pages 45: Heart Rate Variability-based Stress Detection And Fall Risk Monitoring During Daily Activities: A Machine Learning Approach
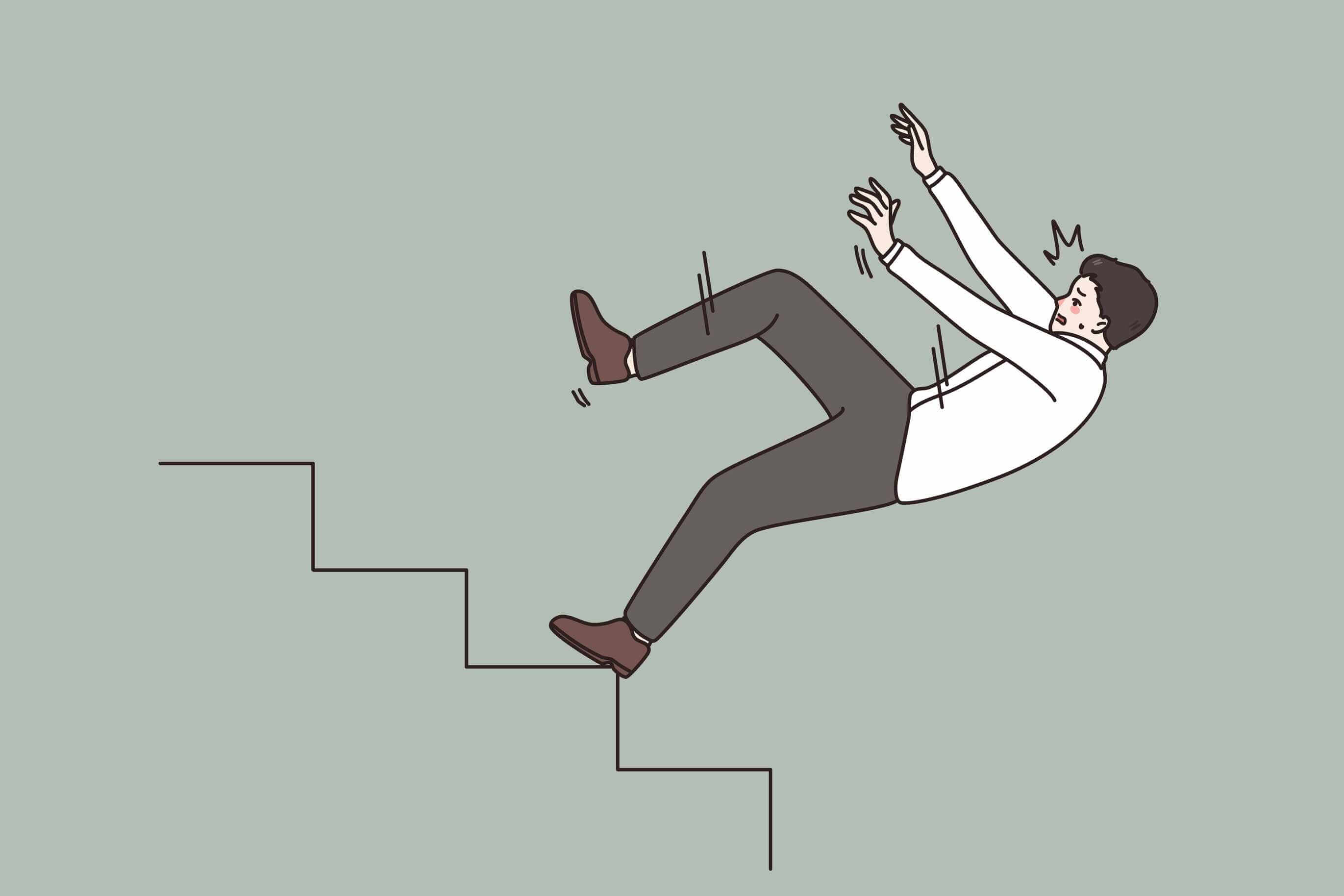
Computers, Vol. 14, Pages 45: Heart Rate Variability-Based Stress Detection and Fall Risk Monitoring During Daily Activities: A Machine Learning Approach
Computers doi: 10.3390/computers14020045
Authors: Ines Belhaj Messaoud Ornwipa Thamsuwan
Impaired balance and mental stress are significant health concerns, particularly among older adults. This study investigated the relationship between the heart rate variability and fall risk during daily activities among individuals over 40 years old. This aimed to explore the potential of the heart rate variability as an indicator of stress and balance loss. Data were collected from 14 healthy participants who wore a Polar H10 heart rate monitor and performed Berg Balance Scale activities as part of an assessment of functional balance. Machine learning techniques applied to the collected data included two phases: unsupervised clustering and supervised classification. K-means clustering identified three distinct physiological states based on HRV features, such as the high-frequency band power and the root mean square of successive differences between normal heartbeats, suggesting patterns that may reflect stress levels. In the second phase, integrating the cluster labels obtained from the first phase together with HRV features into machine learning models for fall risk classification, we found that Gradient Boosting performed the best, achieving an accuracy of 95.45%, a precision of 93.10% and a recall of 85.71%. This study demonstrates the feasibility of using the heart rate variability and machine learning to monitor physiological responses associated with stress and fall risks. By highlighting this potential biomarker of autonomic health, the findings contribute to developing real-time monitoring systems that could support fall prevention efforts in everyday settings for older adults.