Future Internet, Vol. 16, Pages 472: Enhanced Fall Detection Using Yolov7-w6-pose For Real-time Elderly Monitoring
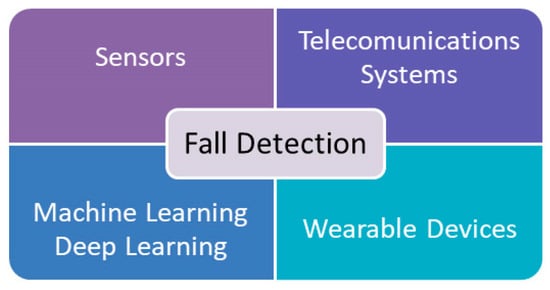
Future Internet, Vol. 16, Pages 472: Enhanced Fall Detection Using YOLOv7-W6-Pose for Real-Time Elderly Monitoring
Future Internet doi: 10.3390/fi16120472
Authors: Eugenia Tîrziu Ana-Mihaela Vasilevschi Adriana Alexandru Eleonora Tudora
This study aims to enhance elderly fall detection systems by using the YOLO (You Only Look Once) object detection algorithm with pose estimation, improving both accuracy and efficiency. Utilizing YOLOv7-W6-Pose’s robust real-time object detection and pose estimation capabilities, the proposed system can effectively identify falls in video feeds by using a webcam and process them in real-time on a high-performance computer equipped with a GPU to accelerate object detection and pose estimation algorithms. YOLO’s single-stage detection mechanism enables quick processing and analysis of video frames, while pose estimation refines this process by analyzing body positions and movements to accurately distinguish falls from other activities. Initial validation was conducted using several free videos sourced online, depicting various types of falls. To ensure real-time applicability, additional tests were conducted with videos recorded live using a webcam, simulating dynamic and unpredictable conditions. The experimental results demonstrate significant advancements in detection accuracy and robustness compared to traditional methods. Furthermore, the approach ensures data privacy by processing only skeletal points derived from pose estimation, with no personal data stored. This approach, integrated into the NeuroPredict platform developed by our team, advances fall detection technology, supporting better care and safety for older adults.