Cognition Chain For Explainable Psychological Stress Detection On Social Media
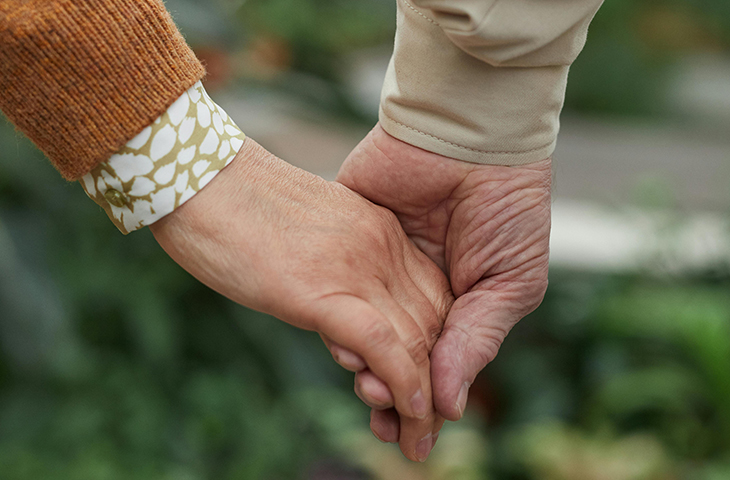
arXiv:2412.14009v1 Announce Type: new Abstract: Stress is a pervasive global health issue that can lead to severe mental health problems. Early detection offers timely intervention and prevention of stress-related disorders. The current early detection models perform "black box" inference suffering from limited explainability and trust which blocks the real-world clinical application. Thanks to the generative properties introduced by the Large Language Models (LLMs), the decision and the prediction from such models are semi-interpretable through the corresponding description. However, the existing LLMs are mostly trained for general purposes without the guidance of psychological cognitive theory. To this end, we first highlight the importance of prior theory with the observation of performance boosted by the chain-of-thoughts tailored for stress detection. This method termed Cognition Chain explicates the generation of stress through a step-by-step cognitive perspective based on cognitive appraisal theory with a progress pipeline: Stimulus $\rightarrow$ Evaluation $\rightarrow$ Reaction $\rightarrow$ Stress State, guiding LLMs to provide comprehensive reasoning explanations. We further study the benefits brought by the proposed Cognition Chain format by utilising it as a synthetic dataset generation template for LLMs instruction-tuning and introduce CogInstruct, an instruction-tuning dataset for stress detection. This dataset is developed using a three-stage self-reflective annotation pipeline that enables LLMs to autonomously generate and refine instructional data. By instruction-tuning Llama3 with CogInstruct, we develop CogLLM, an explainable stress detection model. Evaluations demonstrate that CogLLM achieves outstanding performance while enhancing explainability. Our work contributes a novel approach by integrating cognitive theories into LLM reasoning processes, offering a promising direction for future explainable AI research.