Analytical Ai: A Better Way To Identify The Right Ai Projects
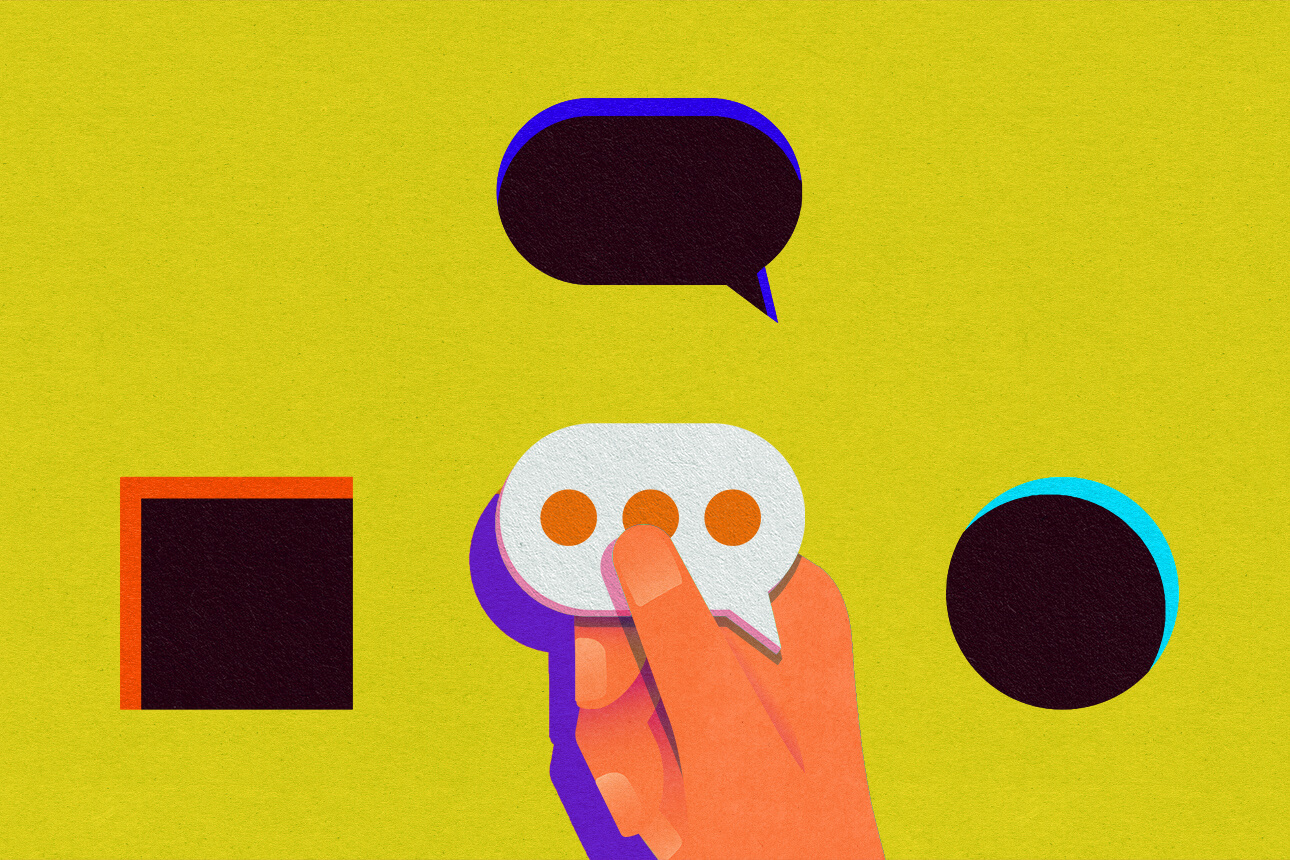
Carolyn Geason-Beissel/MIT SMR | Getty Images
Large language models, with their ability to generate humanlike text and assist in everyday tasks, have made generative AI tools like ChatGPT the poster children of artificial intelligence. However, amid the allure of this innovative technology, there lies a significant risk: the potential overshadowing of advanced analytics and traditional AI — analytical AI, for short. Analytical AI has consistently demonstrated its value in enhancing business decisions and processes, yet it risks being neglected in favor of GenAI’s new and exciting capabilities. According to research from McKinsey, generative AI use cases could add to the economic benefits projected for analytical AI use cases by 15% to 40%. This prediction underscores the need for a balanced approach when deploying AI projects.
Leaders should recognize that generative AI and analytical AI are complementary rather than interchangeable. GenAI focuses on efficiency and automation, like using AI-powered chatbots to increase call center productivity, whereas analytical AI enhances strategic decision-making, like determining the best time or offer for each customer contacted by the call center.
Which flavor of AI suits your business problem best? A critical step in creating a road map for AI projects involves conducting ideation workshops in which business and technical leaders can brainstorm about opportunities to apply AI to organizational challenges. While conducting hundreds of these workshops, we have seen two main difficulties arise: Business leaders often struggle to effectively spot opportunities to apply analytical AI, and technical teams seldom have the necessary business awareness to guide the discussion and influence the chosen projects. The good news is that, just as a GenAI-powered chatbot can benefit call center operations by helping to resolve customer issues more efficiently, a GenAI companion ideation tool can yield substantial benefits during these workshops.
Conducting Ideation Workshops With GenAI
Let’s explore what such ideation workshops should look like. They are typically organized into two stages. First, there’s a divergent stage, in which multiple groups (typically three or four, with six people in each) that comprise multidisciplinary executives and rank-and-file workers identify a broad range of ideas. These ideas usually revolve around specific pain points in existing business processes, such as inefficiencies, bottlenecks, or repetitive tasks; or opportunities for enhancement, like improving customer experiences, increasing operational efficiency, or enabling data-driven decision-making. The ideas are then organized into clusters of related topics, such as customer service or supply chain optimization. In the subsequent convergent stage, each topic cluster is further explored to find AI solutions that fit the business in terms of impact, time to market, and cost.
A generative AI companion tool can be used here to improve the output of both stages across six steps. (You can try out a version of a generative AI companion tool online.)
In the divergent stage, the GenAI tool helps in (1) idea generation, (2) idea structuring, and (3) data exploration. In the convergent stage, it assists in (4) identifying ways in which AI can facilitate idea implementation, (5) developing an intriguing pitch to present the AI project, and (6) quantifying the business or financial impact connected to pursuing the opportunity. At least one member of each group should be familiar with the tool. Moreover, for the tool to be of any help, your team will need to customize the large language model (LLM) as needed.
Customizing the Large Language Model
Before the workshop begins, your team must customize the LLM by feeding the company’s strategic reports, financial records, business process documentation, and the data inventory into it. This way, the LLM is equipped with the necessary context and knowledge about the company’s operations and strategic goals. The data inventory allows the LLM to generate ideas grounded in the organization’s actual data resources.
Another fundamental element of customization is to have your team craft a prompt to instruct the model. The prompt should begin with some context by describing the workshop goals, assigning the LLM the role of an energetic company adviser/data expert, and providing helpful information about the documentation. Furthermore, the crafted prompt should instruct the LLM with contextual answers and promote the model to ask follow-up questions, aiming for clarity.
The teams are now ready to move on to the divergent stage.
The Role of the GenAI Companion Tool in the Divergent Stage
The three steps within the divergent stage involve the following actions:
Idea generation: After deep-diving into the group’s pain points and opportunities, the LLM ensures that no potential opportunity is overlooked, mitigating human biases that lead people to favor certain types of opportunities while disregarding others. For example, rank-and-file stakeholders focus more on identifying efficiency opportunities, while top management tends to explore growth (client-focused) opportunities. The LLM helps answer the question “What other opportunities might exist to use AI in the organization?”
Idea structuring: After the initial individual ideation process, each group member shares the pain points and opportunities that they see for using AI tools. A moderator is responsible for compiling and clustering these ideas in real time, which can be a tricky task. By asking participants to submit their ideas through a digital form, the LLM can answer the question “How should the pain points be structured and clustered?” This approach is valuable because it saves time, eliminating the need for lengthy rounds of explanations from each participant.
Data exploration: The participants in the multidisciplinary groups may spend some time interacting with the LLM to discover the types of data available within the company. The model plays the data expert role, seizing the data inventories it has been fed for this task. This helps answer the question “Which data is available about [area of interest]?” This step proves to be helpful because business stakeholders may not be aware of all of the available data within the company and can be quickly introduced to it via the LLM.
The Role of the GenAI Companion Tool in the Convergent Stage
Once teams have moved through the divergent stage, they’re ready to use the generative AI tool to work through the steps of the convergent stage.
AI solution: In the convergent stage, the LLM plays a different yet valuable role — helping to answer the question: “How can generative and analytical AI help address the pain point/opportunity?” The LLM blends not only contextual information about the company and its data inventory but also its broader knowledge of advanced analytics and other AI techniques, together with industry best practices, to propose realistic options for using AI within the company. This task is particularly important because, as mentioned, business stakeholders often lack the technical and data know-how to discuss these solutions thoroughly.
Pitch design: When it comes to pitching an AI solution for an opportunity, success hinges not just on the idea itself but on how it’s framed and communicated. In these ideation workshops, ideas generated within one group are shared with the other groups. The custom LLM supercharges this task by crafting a compelling and well-organized pitch that resonates with the intended audience. The GenAI tool answers the question “How should the pitch be structured to present the opportunity effectively?”
Opportunity quantification: Finally, every project, whether AI-related or not, requires a business case that quantifies the expected benefits of increasing sales, improving the margin, or optimizing any other relevant metric. While using the LLM to make a direct quantification doesn’t yield the best results — LLMs aren’t particularly good at doing math — the GenAI tool can provide valuable insights on how to estimate potential gains by answering the question “How can we measure the expected benefit of the AI solution?” This guidance is crucial for building a persuasive and well-supported business case.
Lessons From Practice: A Case Study
A holding company that owns businesses in telecommunications, retail, banking, and real estate used a generative AI companion tool in a series of ideation workshops. The company aimed to understand how to exploit advanced data monetization initiatives driven by AI projects that could use data from its multiple companies.
First, the company fed only structured data into the model, which resulted in overly technical responses from the LLM. Next, the company included a detailed textual description of each variable to ensure that the LLM had enough context to generate more insightful and relevant answers. For instance, when prompted to generate ideas for boosting the bakery sales of a retailer, the LLM suggested using consumer and geolocation data to optimize promotion opportunities and choose the most ideal retail locations.
From an organizational perspective, informing participants about the generative AI companion tool ahead of the workshop helped ensure that they didn’t waste time familiarizing themselves with it. When no prior information was given, many groups did not use the full potential of the custom LLM.
The generative AI companion tool played a vital role in data exploration. At holding companies or large corporations, it’s common to find that stakeholders are unaware of the existing data in all subsidiaries or departments. When prompted to talk about the available consumer behavior data, for example, the LLM highlighted a diverse range of data sets, such as the banking business’s data on customer spending habits, customers’ purchase patterns at retail outlets, and telecommunications data, such as mobile usage, geolocation, and browsing history. This overview helped stakeholders identify untapped resources for exploring related business opportunities, such as better customer profiling.
During the idea-generation work, the AI companion proved to be crucial. The team composed of seasoned executives and rank-and-file workers initially showed a clear bias toward customer-facing opportunities, but the LLM helped highlight other interesting opportunities for efficiency improvements. The GenAI tool, for instance, suggested that the retailer could “use telco real-time location to track deliveries, providing greater visibility of the supply chain.”
As we anticipated, some business stakeholders were not at ease using an AI tool to address a pain point. However, with the help of the generative AI companion tool, even not-so-straightforward business and AI opportunities could be addressed. In one workshop, banking representatives wanted to increase home insurance sales. The LLM suggested using retailers’ transactional data for predictive analytics on purchases and deploying GenAI to create personalized marketing campaigns for high-probability buyers.
The LLM proved valuable not only during the workshops for generating AI solutions but also in the follow-up stages, where the team detailed each use case and developed a comprehensive road map of opportunities. Because users left the workshop with a detailed deep dive into each opportunity, their ability to take subsequent steps was significantly improved.
Overall, the outcomes of using this generative AI companion tool were highly effective for the holding company: It identified about 100 possible AI projects, a twofold increase over the company’s expectations based on the number of workshop participants. These projects are projected to deliver millions of euros to the bottom line of the various subsidiaries.
The impact extended beyond quantitative measures to qualitative benefits. Compared with their experience in workshops without a generative AI companion tool, participants tackled a wider diversity of subjects, including customers, operations, and corporate topics. Moreover, people were able to talk in deep, concrete ways about the use cases within the time limits of the workshops.
Three Takeaways for Leaders
By integrating a generative AI companion tool, teams can feel assured that they are not downplaying the potential of GenAI but rather are using it to unearth both analytical and GenAI projects.
Continually reviewing and refining your AI strategies to stay aligned with your company’s business goals is a must. A generative AI companion tool can significantly enhance the effectiveness of ideation workshops, helping them run more smoothly and with more energy. As a result, AI leaders can run these workshops more frequently and independently of large-scale road map exercises — driving more agile and creative outcomes.
Finally, the generative AI companion tool empowers and connects both technical and business teams. By providing each team with the knowledge they are missing, the custom LLM levels the playing field and fosters effective collaboration, which is crucial for successful AI projects.
While generative AI captivates people’s attention, companies should not overlook the proven capabilities of analytical AI. By integrating these tools thoughtfully and strategically, companies can unlock the full potential of artificial intelligence, driving innovation and value creation. For leaders ready to embrace this approach, the future of AI in business is not just bright — it is transformative.