Inverse Reinforcement Learning With Switching Rewards And History Dependency For Characterizing Animal Behaviors
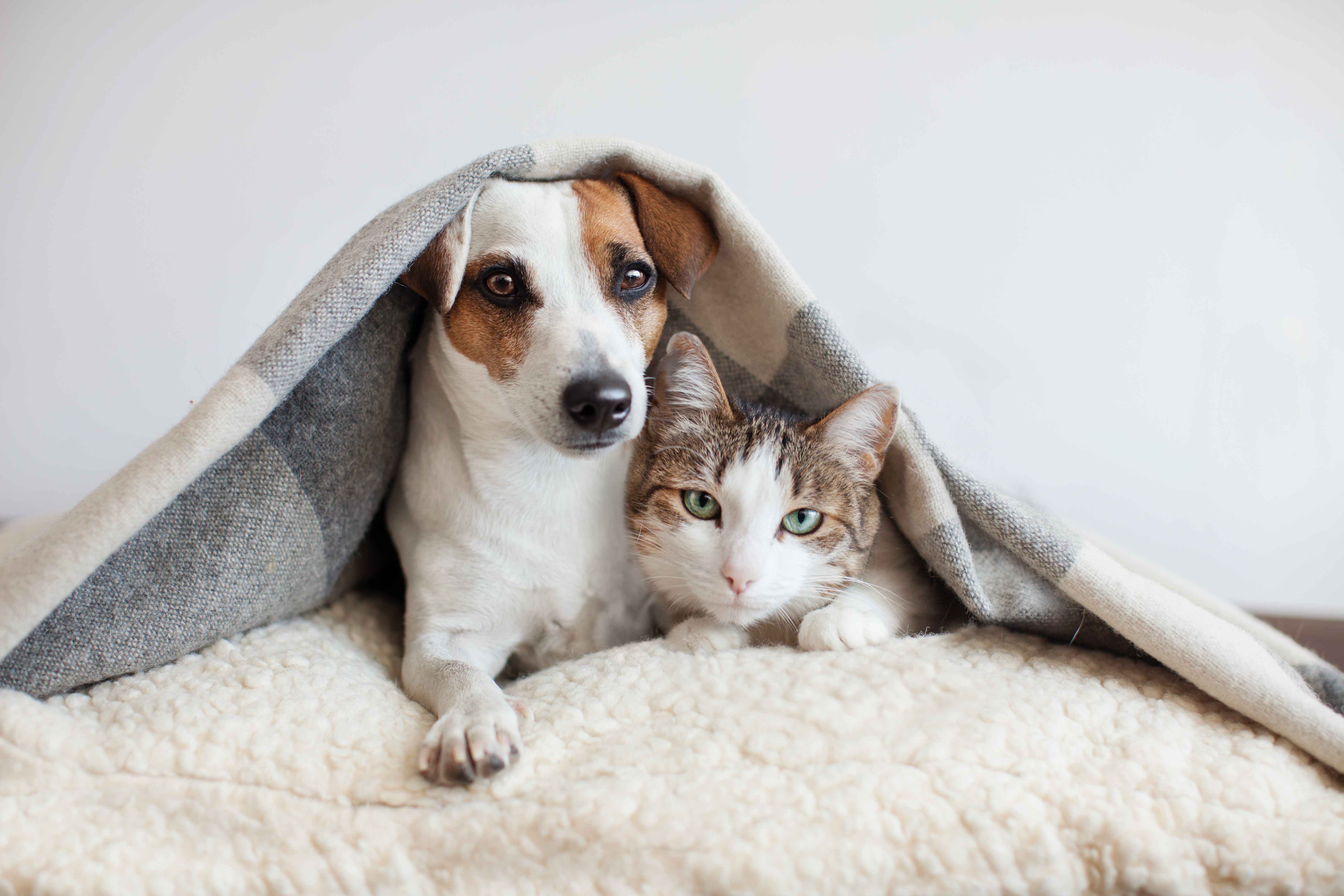
arXiv:2501.12633v1 Announce Type: cross Abstract: Traditional approaches to studying decision-making in neuroscience focus on simplified behavioral tasks where animals perform repetitive, stereotyped actions to receive explicit rewards. While informative, these methods constrain our understanding of decision-making to short timescale behaviors driven by explicit goals. In natural environments, animals exhibit more complex, long-term behaviors driven by intrinsic motivations that are often unobservable. Recent works in time-varying inverse reinforcement learning (IRL) aim to capture shifting motivations in long-term, freely moving behaviors. However, a crucial challenge remains: animals make decisions based on their history, not just their current state. To address this, we introduce SWIRL (SWitching IRL), a novel framework that extends traditional IRL by incorporating time-varying, history-dependent reward functions. SWIRL models long behavioral sequences as transitions between short-term decision-making processes, each governed by a unique reward function. SWIRL incorporates biologically plausible history dependency to capture how past decisions and environmental contexts shape behavior, offering a more accurate description of animal decision-making. We apply SWIRL to simulated and real-world animal behavior datasets and show that it outperforms models lacking history dependency, both quantitatively and qualitatively. This work presents the first IRL model to incorporate history-dependent policies and rewards to advance our understanding of complex, naturalistic decision-making in animals.